Revolutionizing digital pathology through deep learning
In the rapidly evolving landscape of healthcare and medicine, artificial intelligence (AI) and deep learning (DL) technologies are emerging as transformative tools, reshaping the way diagnoses are made and diseases are studied. One area where these new technologies are making a major impact is investigating the digital pathology of brain tumors. Key to these advances is the development of a hybrid model based on YOLOv5 and ResNet50 for accurate tumor localization and predictive grading within histological whole slide images (WSIs).
A new approach to brain tumor diagnosis
The traditional process of diagnosing brain tumors has been largely based on histopathological examination, which is complex, labor-intensive and requires specialized analysis. However, AI models armed with deep learning capabilities promise to significantly reduce the burden on pathologists. Recently, a new technique has been developed that leverages the hybrid network YOLOv5 and ResNet50 to visualize predictive grading of brain tumors on histopathology images.
This technique has proven effective in identifying brain tumors and estimating glioma grades, superior to current methods. It provides competitive performance in classification of four classes of glioma, which greatly impacts the discrimination between tumor subtypes. The proposed hybrid model ensures stable training dynamics and robust model performance, making it a promising development in the field of digital pathology.
Addressing challenges in pathological anatomical imaging
One of the major challenges in digital pathology is dealing with high-dimensional pathological anatomical images. These images require advanced techniques for accurate analysis and diagnosis. AI models, especially those based on deep learning, have shown superior performance in this regard. They have shown promising results in creating a new computer-aided diagnosis (CAD) system based on transfer learning for the diagnosis of healthy grading and glioma.
The proposed CAD system combines YOLOv5 and ResNet50 models, providing a comprehensive model for accurate classification and diagnosis accuracy. This system not only enhances the accuracy and robustness of brain tumor classification, but also mitigates the risk of overfitting, a common problem in machine learning.
Deep learning for brain tumor localization and classification
A robust method for multi-type classification of brain tumors using deep fusion is proposed. This method is based on convolutional neural networks and achieves a classification accuracy of 99.18% and 97.24% on the Figshare dataset and Kaggle dataset, respectively. The proposed model integration techniques offer the potential to overcome the limitations of relying on a single model, paving the way for the diagnosis of advanced brain tumors.
Enhancing the interpretability of models
As the application of deep learning for brain tumor localization and classification becomes more widespread, it is crucial to understand the interpretability of these models. This understanding will provide insight into how these models classify brain tumors, thus enhancing transparency and trust in these AI systems. As this field continues to grow, exploring interpretability in deep learning models will become increasingly important in identifying and classifying brain tumors using MRI images.
Conclusion: The future of brain tumor diagnosis
The use of deep learning techniques in digital pathology investigations of brain tumors represents a major step towards a future in which accurate and efficient diagnosis is the norm. The development of hybrid models such as YOLOv5 and ResNet50, combined with the power of AI and deep learning, is expected to revolutionize the field of digital pathology, leading to faster, more reliable diagnoses and ultimately better patient outcomes.
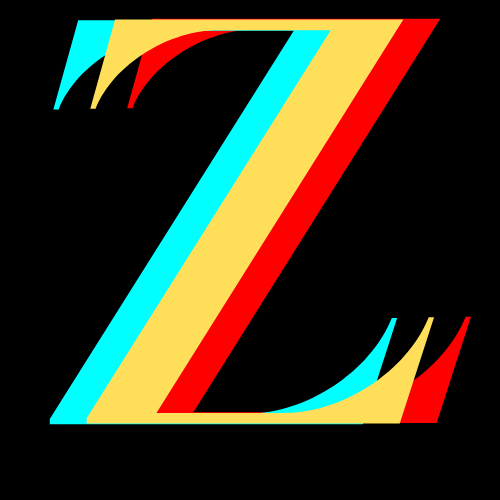