Unconscious biases such as first impressions and gut feelings influence hiring practices. For example, research shows that labor markets discriminate based on people’s names. Stereotypical white-sounding names received 50 percent more interview callbacks than African names. The research concludes that if we accept unequal labor market outcomes, “Emily and Greg are more employable than Lakeisha and Jamal.”
It is clear that cognitive biases are prevalent in the hiring process. According to psychologists, the halo effect is the most common cognitive bias in hiring decisions. This is where the recruiter focuses on one positive aspect of the candidate in making a general judgment. For example, the halo effect can cause outwardly attractive people to get jobs at the expense of others. Confirmation bias is another type of cognitive bias. It describes the tendency to interpret information in a way that confirms one’s beliefs. Therefore, if a recruiter has an initial perception of a candidate, he or she will look for information that supports that perception. Unfortunately, if initial perceptions are tainted by sexual, racist, or homophobic thinking, the hiring manager may try to find a way to confirm his or her existing bias in hiring candidates.
Can AI end unconscious bias in the hiring process?
For these reasons, many believe that artificial intelligence (AI) should be introduced into the hiring process to reduce unconscious bias.
The use of artificial intelligence in the recruitment process has become commonplace. In the UK, 58% of recruiters rely on AI when recruiting. This is because AI has the potential to reduce human bias and uncover hidden talents that traditional hiring practices ignore. The AI can be programmed to ignore demographic information about candidates, such as race, gender, gender, and zip code. Instead, candidates can be judged on their skills and experience alone, while human managers can never separate a person from their resume. In this regard, AI may help an organization to recruit a more diverse workforce.
However, this ignores an important fact: AI is fed information by humans in order to learn and produce algorithms — and human information contains biases that can lead to discriminating algorithms. Algorithms built on historical datasets of successful applicants are a perfect example of this. The historical datasets contain decades of discriminatory hiring practices, so “the historical dataset of successful applicants will essentially be a male-dominated dataset.”
The implications of this were seen in 2014 at Amazon when a group of engineers in Scotland set up a program to recruit top talent. However, because the algorithm was trained on previous successful apps, it favored men over women. The algorithm learned to screen applicants by penalizing words like “women’s chess club” and downgrading candidates from all-women’s colleges.
In 2017, Amazon abandoned the recruitment tool. The failed experiment left tech experts worried that AI would not remove human biases, but rather automate them. Caroline Criado-Perez, author Invisible women: Exposing data bias in a world designed for men. He argues that algorithms can actively work to amplify biases. Self-learning algorithms can learn to confirm and amplify what they already know. In other words, if an algorithm is sexist, it can teach itself to become more sexist. A University of Washington study showed how an algorithm learned to associate women with pictures of the kitchen because women were 33% more likely to be seen in pictures in the kitchen than men. The algorithm increased this disparity to 68%, by classifying men as women simply because they were standing next to dirty dishes. It is clear that machine learning can amplify gender biases, and reinforce social and economic divides in the workplace.
How can we prevent the algorithm from being favourited?
Technology is here to stay. Companies are keen to maintain algorithmic tools to reduce time and expense in the hiring process. So, how can we mitigate algorithm biases?
One of the advantages of using algorithms over human recruits is that algorithmic bias can, in principle, be identified and corrected. But correcting algorithmic bias might mean changing the datasets we give them, or actively teaching them to remove biases. A company called Pymetrics aims to make this happen. Pymetrics uses a neuroscience-based game that measures risk aversion. The company’s top performing players complete the game and an algorithm is produced to detect key traits of successful workers. The recruiter can then compare the candidates to the company’s best performing employees. Therefore, graduates who may be fortunate enough to work as apprentices without pay, study abroad, or use parental connections have no advantage over their classmates.
Unfortunately, such algorithms are the exception rather than the rule. In order to remove biases, operators need to actively address the factors that contribute to them. The tech companies creating the algorithmic architectures of the modern workplace certainly have a long way to go. In 2016, it was found that ten Silicon Valley companies did not employ a single black woman, three companies had no black employees at all, and six had no women at the executive level. This severe lack of diversity means that their employees are less likely to understand certain biases, let alone mitigate them.
Algorithms are in part “our opinions embedded in the code”. Until more efforts are done to mitigate data biases, we should not hold out hope that AI can help correct biases in the labor market and workplace.
Emily Skinner is a Research Associate with the Bristol Model Project at the University of Bristol.
Image credit: Magnet.me on Unsplash
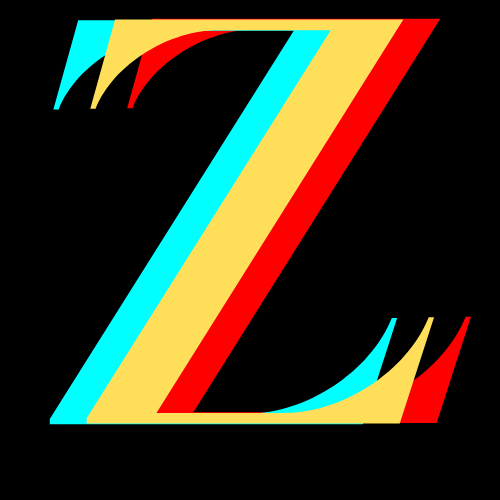